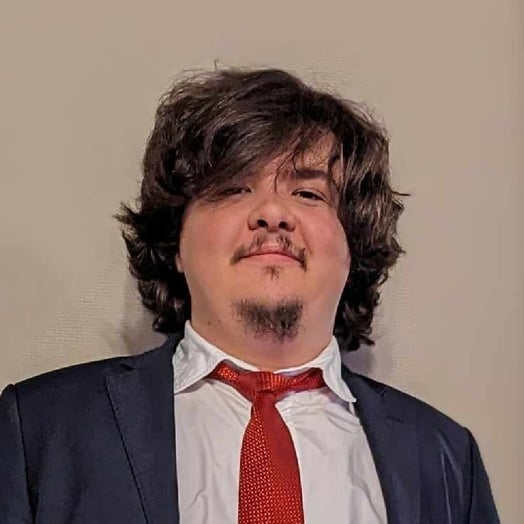
Andrei Apostol
验证专家 in Engineering
人工智能开发人员
Andrei studied computer science in his hometown in Romania and completed his master's degree in AI at the University of Amsterdam. 经过多年的训练,他积累了人工智能方面的实践经验, 开发数据处理管道, and deployment. 他是一名工程师,总是期待新的挑战. Andrei also has academic experience through publishing two papers on neural pruning and quantization, 哪些受到了学术界的好评.
Portfolio
Experience
Availability
首选的环境
Linux, Visual Studio Code (VS Code), Slack, Rocket.聊天,谷歌云/套件
最神奇的...
...thing I've accomplished is earning the Best Paper award for publishing my master's dissertation in BeneLearn 2020 about a novel pruning algorithm I developed.
Work Experience
机器学习工程师
Omnimodular
- Built a custom and flexible BERT-like architecture for multi-class document classification and trained on data from various clients, 获得90-94%的平均准确率.
- Combined the traditional NLP augmentation model with the GPT-3 large language model to do data augmentation for clients, 将错误率降低50%.
- Used Hugging Face datasets based on Apache Arrow to handle large volumes of data that normally would not fit in memory and implemented an efficient and replicable data processing pipeline with batching and multiprocessing.
- 定期召开客户会议, giving high-level overviews of our technical solution and explaining our core metrics.
机器学习工程师
Braincreators
- Trained a YOLOv5 object detection network for waste management and recycling, obtaining mean-average-precision scores of over 95% on over 40 classes of objects with a speed of over 200 frames per second on a conventional GPU.
- Built an app around the object detection network using FastAPI to expose the endpoints and Streamlit for the UI, converting the network to the ONNX format after training for faster inference time.
- Created a module for out-of-distribution detection using the CLIP pre-trained model, obtaining over 97% accuracy in the in-/out-of-distribution classification while allowing the class taxonomy to be changed without re-training.
- 与项目干系人定期举行会议, led demos and presentations for the client to provide estimates for the next milestones, 并进行冲刺计划会议, 跟踪进度.
- Published the paper "Highlights of AI Research in Europe" in a special edition of the European Journal of AI, demonstrating that pruning and quantization can bring greater acceleration when used without sacrificing accuracy.
- Implemented object tracking using the SORT algorithm with re-identification to follow the trajectories of objects over time. 获得85%以上的MOTA(多目标跟踪精度)和80%以上的IDF1.
机器学习工程师
Mantis NLP
- Designed a BERT-based architecture for medical research document tagging. 实施有效和可扩展的培训管道, 能够在几个小时内处理1500多万份文件. 达到了最先进的微f1分数的70%.
- 创建了一个易于创建的面向用户的应用程序, deployment, and removal of machine learning models in production using AWS Sagemaker. Included monitoring, alerts, and a complete test suite to ensure quality and reliability.
- 使用Langchain与ChatGPT和FAISS. Created a personal assistant that could answer a user's questions based on their collection of notes. 执行快速工程以获得更高质量的响应.
- Held close contact with key clients and stakeholders to ensure we were aligned on requirements and created the highest quality deliverables at all stages of the project.
机器学习研究实习
BrainCreators
- Researched and built expertise in neural network pruning techniques as part of my master's dissertation.
- Developed a novel pruning algorithm that obtains state-of-the-art results for high sparsity scenarios and other properties such as the ability to prune during training, 计算温顺, 以及超参数不变性.
- Received the Best Paper award for writing a scientific paper around said algorithm and publishing it at the BeneLearn 2020 conference held in Belgium, Netherlands, and Luxembourg.
Data Scientist
FeedbackFruits
- 利用SARIMA方法建立了时间序列预测模型, achieving a low mean square error for all predictions within the confidence bound.
- Created an encoder/decoder gated recurrent unit network for document part classification, 获得超过90%的准确度.
- Deployed the trained models to production by exposing the core functionality via RESTful APIs and monitored the performance in production.
研究科学家实习生
Amazon.com
- Analyzed customer behavior on the platform and developed a random forest model with a high ROC-AUC score.
- Handled large volumes of data using Apache Spark and created data processing pipelines to filter and prepare data using the Python and Scala APIs and Spark SQL.
- Conducted A/B testing and integrated the resulting model into several Amazon sites.
Experience
FlipOut |通过符号翻转发现冗余权重
http://github.com/AndreiXYZ/flipoutIt can remove over 98% of the connections in common networks with little to no impact on accuracy, 允许大的速度增益. 与文献中的基线相比, 这种方法可以在训练时进行修剪, 对超参数的选择不敏感, 并且允许直接选择稀疏度级别.
I wrote a paper around this method and published it in BeneLearn 2020, 获得最佳论文奖.
Education
人工智能硕士学位
阿姆斯特丹大学-阿姆斯特丹,荷兰
计算机科学学士学位
亚历山德鲁伊安库萨大学- Iași,罗马尼亚
Certifications
模型并行:构建和部署大型神经网络
NVIDIA
雅思学术证书(母语水平)
英国文化协会
Skills
Libraries/APIs
PyTorch, Matplotlib, OpenCV, Scikit-learn, Pandas, PyTorch闪电, TensorFlow, NumPy
Tools
TensorBoard, 你只看一次(YOLO), Slack, GitHub, ChatGPT, Git, Docker Compose, Spark SQL, 亚马逊SageMaker
Frameworks
Flask, Streamlit, Apache Spark
Languages
Python 3, Python, Bash, SQL
Platforms
Visual Studio Code (VS Code), Rocket.聊天、Linux、Docker、齐柏林飞艇、AWS IoT、亚马逊网络服务(AWS)
Paradigms
面向对象编程(OOP),数据科学,敏捷,REST
Other
机器学习, Deep Learning, 自然语言处理(NLP), 计算机视觉, 长短期记忆(LSTM), 深度神经网络, 神经网络修剪, Quantization, FastAPI, 对象检测, Transformers, BERT, Classification, English, 谷歌云/套件, 人工智能(AI), 概念验证(POC), 最小可行产品(MVP), 自然语言理解(NLU), Contract, 信息提取, 生成预训练变压器(GPT), OpenAI GPT-3 API, 数据可视化, Statistics, 软件工程, 科学数据分析, Random Forests, APIs, 科学计算, Research, NVIDIA Triton, 检测工程, 开放神经网络交换(ONNX), Hugging Face, DVC, 机器学习操作(MLOps), 语言模型, Algorithms, Data Analytics, 大型语言模型(llm), 英伟达TensorRT, 图像处理, 生成对抗网络(GANs), 生成式人工智能(GenAI), OCR, PDF, Chatbots, OpenAI API, 信息检索, 集群计算, Time Series, ARIMA Models, DeepSpeed, 3D, OpenAI GPT-4 API, LangChain, FAISS, 生成预训练变压器3 (GPT-3), 提示工程
如何使用Toptal
Toptal matches you directly with global industry experts from our network in hours—not weeks or months.
分享你的需求
选择你的才能
开始你的无风险人才试验
对顶尖人才的需求很大.
Start hiring